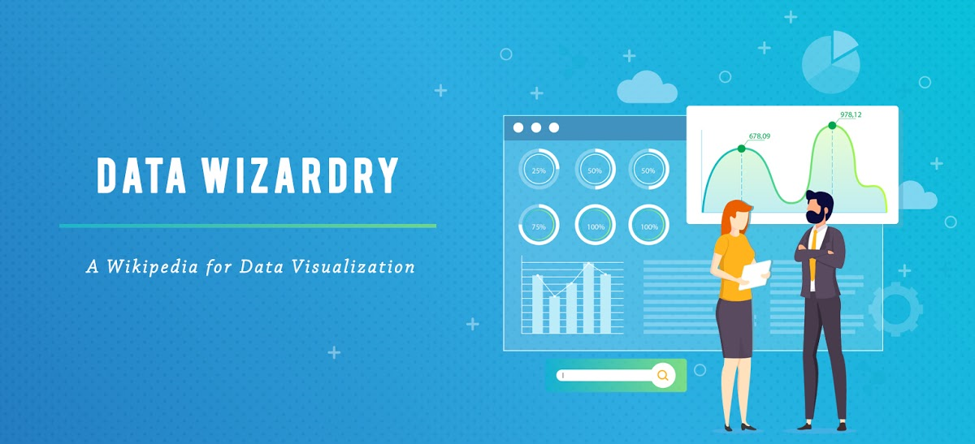
Data Wizardry – A Wikipedia for Data Visualization
Data Wizardry – A Wikipedia for Data Visualization
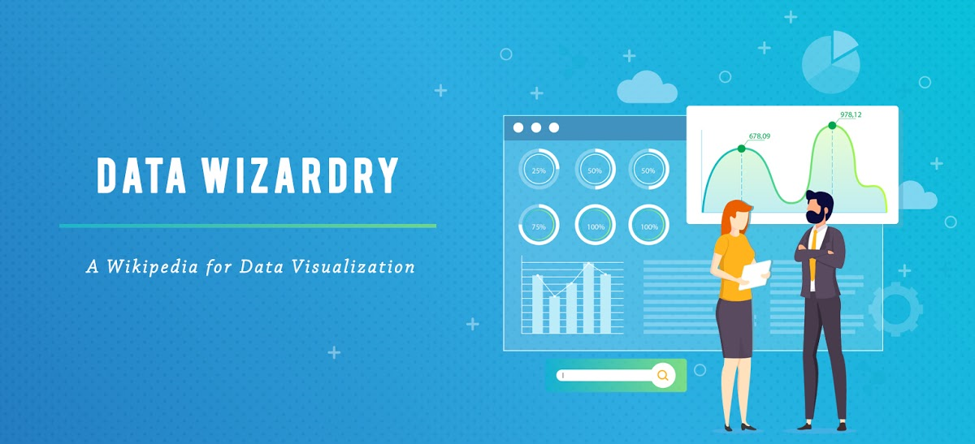
“The greatest value of a picture is when it forces us to notice what we never expected to see.” - John Tukey
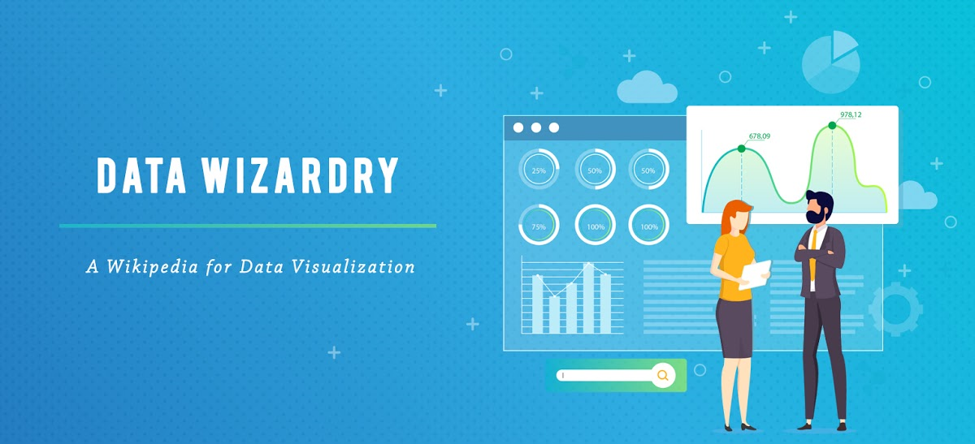
By Ranjith Udayakumar, Data Science & ML Practitioner with a pinch of Cloud and RPA Development Expertise
There is a saying, you may have heard multiple times - “Data is the new oil!”. Now, it’s true, Data became the new oil to the world but unlike oil Data is an abundant, valuable and powerful commodity which brings out hidden insights onto the table when it is analyzed and visualized in a meaningful way.
Since data visualization is the last phase in the process of “Data Science” people will often focus less in this phase, but it is essential to narrate a compelling data story and puts out the facts in a way that captures people’s senses in the nick of time.
In this article, I will walk you through the patterns and relationships that make data meaningful and enlightens us to extract the insights we intended to get out of the data, how to choose the right visual for your data.
Is This Article for Me?
This article is meant for everyone! This includes students who just want to familiarize with general concepts of data visualization, professional data analysts and data scientists who want to leverage data visualization techniques and tools to extract insights and actionable information and to narrate visually compelling stories.
Overview of the article
Your data is only as good as the ability to understand the underlying patterns and relationships in the data, communicating it to a larger audience in a visually appealing manner which is why understanding the data and choosing the right visualization for your data is essential.
Data Flavours:
There are two flavours of data, at a very high level, when you are measuring something and giving a numerical value to it, you are creating a quantitative data. when you are grouping something according to categorical value, you are creating a qualitative data But there are different types of quantitative and qualitative data.
Quantitative Data: Discrete and Continuous
Data that can be counted or measured are Quantitative Data. It can be Discrete or Continuous Numerical Values.
-
Discrete Data is a numerical value that is considered as whole indivisible entities.
(i.e.) Values that can’t be more precise. For instance, the number of stores, 47.
-
On the other hand, Continuous Data is a numerical value that could be divided to get finer details.
(i.e.) Values that can be more precise. For instance, Revenue over year, 32M.
Qualitative Data: Binominal, Un-ordered, Ordered
Data that can be grouped according to the category are Qualitative Data also called Categorical Data.
-
Binomial Data: Take on only two possible states.
For instance Accept or Reject, it either takes Accept or Reject as value.
-
Ordered Data: Categories that take implicit or natural order.
For instance Short, Medium, Tall, it follows some order like Small—>Medium—>Tall.
-
Un-Ordered Data: Categories that don’t take implicit or natural order.
For instance India; China; Japan; USA, it doesn’t follow in any specific order.
Data Patterns:
Data is not only growing, but it is also changing faster than before. When it comes to analyzing the data, identifying and exploring the data patterns such as trends, correlations and outliers will help us to find the insights hidden in the data. Initially, it may seem overwhelming, but it helps to harness analytics to understand customer behavior and gives business a competitive edge over others.
Trends:
The pattern of gradual change of data points over time.
For instance, Revenue over Time.
Correlations:
The pattern of association of a data variable with other data variables.
For instance, Revenue vs Customer Satisfaction
Outliers:
The pattern of how some data points are distant from other similar points.
For instance, Revenue from the unusual region.
Data Relationships:
Understanding the relationships exists between the data variables will enable us to derive the intended insights out of data with the help of appropriate visualization.
Nominal Comparison:
Comparison of the numerical values of categories.
For instance: Revenue by Store
Part-to-Whole:
A sub part of data compared to the larger whole data.
For instance: Revenue by Product Segmentation
Time Series:
Changes in data points over time.
For Instance: Monthly Revenue
Distribution:
Value distribution around a center value.
For instance: Revenue by Year
Correlation:
Association between two or more variables.
For instance: Revenue vs Customer Satisfaction
Deviation:
How far any data points from the mean.
For instance: Revenue on Normal days vs Double11.
How to choose the right visualization for your data?
No matter who you are or what you are trying to achieve with the data you have, chances are, there will be times you have to use your data to effectively present the insights that you have derived from the data to inform and influence the business decisions.
If your data story is misinterpreted or not properly conveyed and presented, then your audiences may lose the key insights. That’s why telling compelling data story hidden behind those numbers is undisputed. The ability to understand the data and communicate the data story behind the data is a learned skill, we are here going to see how to hone that skill.
To compare data values:
The following charts are extremely helpful when you want to display measures compared by its magnitude.
-
Bar (Highly Preferred when data points are between 5-15)
-
Radar
-
Funnel
-
Table
-
Pivot Table
-
Polar
-
Tornado
-
Word Count
To show the composition of something:
The following charts are extremely helpful when you want to show how individual data points make up the whole of something.
-
Pie (Highly Preferred when data points are less than 5)
-
Stacked Bar (Highly Preferred when data points are between 5-15)
-
Area
-
Tree Map
To understand the distribution of your data:
The following charts are extremely helpful when you want to understand the outliers and distribution of data points in your dataset.
-
Scatter Plot (Highly Preferred when you have two measures and one categorical value)
-
Cat. Scatter Plot
-
Line
-
Bar
To analyze trends in your data set:
The following charts are extremely helpful when you want to understand how the progress of data indicator over time in your dataset.
-
Line
-
Dual-Axis Line
-
Bar
To better understand the relationship between value sets:
The following charts are extremely helpful when you want to understand how one variable relates to one or numerous different variables.
-
Scatter Plot
-
Bubble
-
Line
-
Conversion Path
To better understand the distribution range across geography:
The following charts are extremely helpful when you want to display the relevant data and distribution range for a country or region on the map.
-
Geo Map (Range)
-
Geo Map (Bubble)
Summary
I hope that this article gives you a better grasp of data flavours, data patterns and relationships and how to choose the right visual for your data when you are analyzing it to transform into insights.
We at OneGlobe have helped our clients across the globe with end to end Data solutions and have incorporated data visualization best practices to leverage a data driven business culture which would enable them with insights to respond to their business and customer needs more quickly and effectively. To know more about our Data Analytics services please visit https://www.oneglobesystems.com/advanced-business-analytics
Leave your thought here
Your email address will not be published. Required fields are marked *